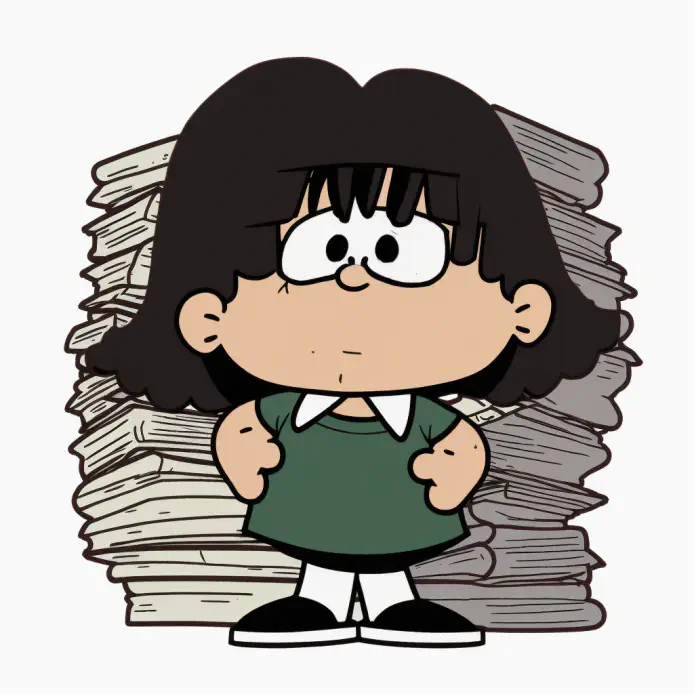
Introducing MAFALDA: A New Benchmark for Fallacy Detection in Natural Language Processing
The ability to accurately detect and classify logical fallacies in text is crucial for various applications in natural language processing (NLP), ranging from improving argumentation quality in debates to combating misinformation online. In this blog post, we introduce MAFALDA (Multi-level Annotated Fallacy Dataset), a comprehensive benchmark for fallacy classification that aims to unify and enhance the existing efforts in this field.
What is MAFALDA?
MAFALDA is a newly proposed benchmark designed to address the fragmented nature of previous datasets on fallacy detection. It consolidates various datasets into a single, more comprehensive collection with a refined taxonomy that aligns, consolidates, and standardizes the classifications of fallacies. This taxonomy not only enhances the consistency of fallacy classification but also makes the process more intuitive and applicable to real-world texts.
Key Contributions of MAFALDA
- Unified Taxonomy: MAFALDA introduces a taxonomy that aligns and refines previous classifications, creating a standard that can be widely adopted for training and evaluating models.
- Manual Annotations: The dataset includes detailed manual annotations for 200 texts, providing clear examples of various fallacies. Each annotation includes a manual explanation, which helps in understanding the rationale behind the classification.
- Subjective Annotation Scheme: Recognizing the subjective nature of fallacy identification, MAFALDA incorporates an annotation scheme that allows for multiple correct labels for certain fallacies, reflecting the real-world complexity of language and argumentation.
- Comprehensive Evaluation: The benchmark evaluates several state-of-the-art language models under a zero-shot learning setting as well as human performance, providing insights into current capabilities and limitations in automatic fallacy detection.
Importance of Fallacy Detection
Logical fallacies are errors in reasoning that weaken arguments. They are frequently used in persuasive language to influence public opinion or diminish opposing viewpoints without engaging logically. Detecting these fallacies is vital for maintaining the quality of discussions and debates, especially in public forums and social media, where misinformation can spread widely.
Performance Insights
Initial evaluations on MAFALDA indicate that while modern language models show promise in detecting straightforward fallacies, they struggle with more nuanced or complex logical errors. This highlights the ongoing challenge in NLP to handle the subtleties and complexities of human language.
Future Directions
With MAFALDA, future research can explore more sophisticated models and techniques for fallacy detection, including few-shot learning and the use of advanced NLP tools. The benchmark itself can be expanded with more annotated examples to cover a wider array of fallacies and scenarios.
Conclusion
MAFALDA represents a significant step forward in the field of computational argumentation, providing researchers and practitioners with a robust tool to improve the automatic detection of logical fallacies. By fostering more nuanced understanding and detection capabilities, MAFALDA contributes to the broader goal of enhancing the quality and reliability of information across various media.
For more information and access to the dataset, visit the MAFALDA GitHub repository.
We hope this benchmark will not only advance the state of research in fallacy detection but also contribute to more rational and factual discourse in public debates.
To cite this paper:
@article{DBLP:journals/corr/abs-2311-09761,
author = {Chadi Helwe and
Tom Calamai and
Pierre{-}Henri Paris and
Chlo{\'{e}} Clavel and
Fabian M. Suchanek},
title = {{MAFALDA:} {A} Benchmark and Comprehensive Study
of Fallacy Detection and Classification},
journal = {CoRR},
volume = {abs/2311.09761},
year = {2023},
url = {https://doi.org/10.48550/arXiv.2311.09761},
doi = {10.48550/ARXIV.2311.09761},
eprinttype = {arXiv},
eprint = {2311.09761},
timestamp = {Tue, 21 Nov 2023 13:55:21 +0100},
biburl = {https://dblp.org/rec/journals/corr/abs-2311-09761.bib},
bibsource = {dblp computer science bibliography, https://dblp.org}
}